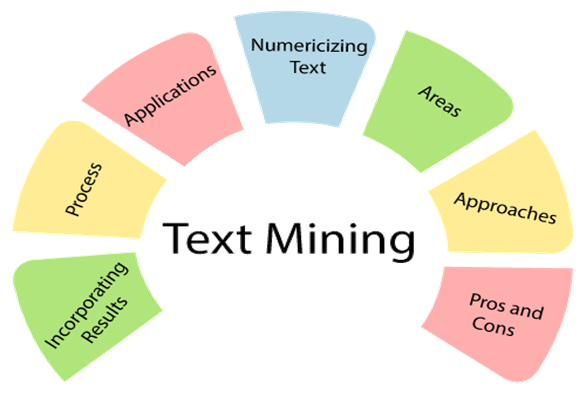
Text Analytics
Text Analytics: Unlocking the Power of Human Language
Introduction to Text Analytics
Text Analytics is the process of extracting knowledge and insights from textual data.
This vast field encompasses a range of techniques, from simple keyword searches to complex machine learning models.
It’s a crucial component in understanding customer sentiment, market trends, and a myriad of other areas where textual data holds significant value.
Text analytics, fundamentally, involves making sense of the patterns, relationships, and insights hidden within human language.
The potential applications of Text Analytics are almost limitless, allowing us to extract knowledge from countless documents, reviews, social media posts, and more.
What exactly is Text Analytics?
Text Analytics goes beyond simple keyword identification.
It leverages sophisticated methods to understand the context, sentiment, and intent expressed in the text.
Think sentiment analysis, topic modeling, and named entity recognition – these are just some facets of Text Analytics.
By using powerful algorithms, Text Analytics helps us bridge the gap between raw text data and actionable insights.
Proper text analysis often requires a nuanced approach tailored to each specific context and intended goal.
Understanding the Need for Text Analytics
How Text Analytics Transforms Businesses
Source: javatpoint.com
In today’s data-rich world, companies generate massive volumes of text data from various sources, including customer feedback, social media posts, and internal communications.
This deluge of information can be overwhelming to process manually.
This is precisely where the transformative power of Text Analytics comes into play, efficiently uncovering hidden patterns within the data.
Text Analytics provides the capability to discern meaningful insights about consumer behaviour, competitive analysis and the overall sentiment surrounding products or services.
The Rise of Big Text Data
With the explosion of social media and online interactions, businesses and researchers find themselves swamped with an incredible amount of unstructured text data.
Manually processing this text would be impractical and unsustainable.
That’s why sophisticated Text Analytics methods have become increasingly critical for leveraging the wealth of insights trapped within these text repositories.
Text Analytics methodologies often involve intricate steps tailored to suit specific scenarios and yield relevant outputs for problem-solving and improved decisions.
Common Text Analytics Techniques
Source: techtarget.com
Natural Language Processing (NLP)
NLP plays a critical role in Text Analytics.
Natural Language Processing techniques equip computers to understand, interpret, and manipulate human language.
It breaks down complex texts into smaller components allowing computers to make meaning from the context and the specific text.
Different NLP models have distinct approaches.
For example, one model could highlight key words, while another extracts meaningful topics.
This diverse application highlights the versatility of NLP methodologies within Text Analytics processes.
Machine Learning in Text Analytics
Advanced Text Analytics often incorporates machine learning algorithms.
Algorithms like Support Vector Machines (SVMs), and Naive Bayes classifiers assist in analyzing huge amounts of data.
Such sophisticated machine learning-driven Text Analytics helps to make connections, predictions and recommendations in a remarkably short span of time.
The applications for Text Analytics using machine learning range from sentiment analysis to content classification.
How to Implement Text Analytics Solutions
Source: repustate.com
Data Preparation for Text Analytics
Before embarking on a text analysis journey, the text data needs careful preparation.
Cleaning the data, standardizing it, handling missing values and formatting inconsistencies are often the preliminary stages to unlock effective text analytics solutions.
Effective text analysis hinges on comprehensive preparation methods.
Choosing the Right Tools for Text Analytics
Various tools are available for Text Analytics projects, offering different functionalities and price points.
Deciding on the correct Text Analytics tools is key for achieving accurate analysis and outcomes.
Consider tools specific to Text Analytics which match your project requirements.
For example, for simple analysis, a cloud based tool could be ideal; while advanced models may require a specialized Text Analytics platform.
Applications of Text Analytics
Sentiment Analysis using Text Analytics
Sentiment analysis – another prominent application of Text Analytics – is used to identify emotions, opinions, and attitudes expressed in texts, using natural language understanding to accurately decode these inputs into meaningful and measurable factors.
Text analytics can measure consumer attitudes about brands, gauge public perception about government actions, or even scrutinize financial sentiment, in just about any data repository containing textual content.
Topic Modeling in Text Analytics
Discovering underlying topics or themes within large corpora of texts is another potent Text Analytics method.
Understanding recurring themes and subject matter, as extracted via effective text analytics can reveal underlying connections that otherwise remain hidden.
Challenges in Text Analytics
Dealing with Noise in Data
Text data is often noisy with irrelevant information.
Efficient noise removal, a crucial step within effective text analytics approaches, needs to happen.
Efficient noise reduction through filtering and other relevant text analytic methods help enhance model accuracy and decision-making.
Handling Large Datasets
The exponential growth in textual data poses challenges.
Robust infrastructure is vital for effective management of massive textual datasets and successful application of relevant Text Analytics methods and systems.
Ethical Considerations in Text Analytics
Privacy and bias are major ethical concerns for many text analysis initiatives.
Developers have a responsibility to craft text analytic processes that adhere to appropriate ethical principles and standards.
It’s equally vital to identify and counteract possible biases during every text analytic stage.
Conclusion: The Future of Text Analytics
Text analytics continues to be a fast-growing and indispensable aspect in business intelligence and data mining.
The applications, possibilities, and tools available to Text Analytics professionals are poised to reshape our future as we understand textual communication more comprehensively than ever before.
Further Questions
Source: sas.com
What are the most relevant applications of Text Analytics in healthcare?
What are the leading Text Analytics technologies being used in education?
Source: futransolutions.com
How is Text Analytics changing customer relationship management (CRM)?
How can I improve my existing Text Analytics procedures for my company?
Can you demonstrate Text Analytics’ effect on news reporting, including bias detection?
These questions demonstrate a comprehensive application-focused Text Analytics approach.
Where does AI come in to text analytics?
AI and Text Analytics are intertwined; AI often powers many Text Analytics capabilities.
The synergy further enriches outcomes, enhancing speed and accuracy.